Explore the key ethical risks AI poses. Gain insights into real-world examples and learn how to navigate these challenges in an evolving legal landscape.
Introduction
In the previous article, Deep Lex explored how AI’s rapid evolution gives rise to fundamental ethical questions related to AI development and use1— questions which jurisdictions around the world are working to address through the introduction of new laws and policy. We discussed how ethics is shaping the framework for AI governance globally and considered the divergent approaches to AI regulation by the EU, US, and China, with key implications for legal professionals.
This article goes a step further to introduce the myriad ethical issues in AI development, providing a roadmap to the forthcoming Deep Lex Ethics Series. We will cover critical issues like bias and discrimination, accountability and transparency, and explore the impact of navigating these challenges.
As we delve deeper into the implications of AI ethics, it becomes clear that understanding these principles is vital for legal professionals who are at the forefront of this rapidly evolving landscape.
Why AI Ethics Matter for Legal Professionals
AI ethics is no longer a theoretical concern but a practical priority. Understanding AI ethics is therefore essential for legal professionals, both conceptually and practically, as it has real-world implications for client risk, regulatory compliance, and professional standards. From automated decision-making in criminal justice to AI-driven hiring, ethical challenges directly impact clients and communities. Addressing risks like algorithmic bias and data privacy can strengthen client trust and shape legal outcomes, underscoring the critical role of the legal profession in responsible AI governance. Conversely, missteps in AI governance can expose clients to liability, jeopardise data privacy, and erode public trust—outcomes that impact client relationships and the integrity of the legal profession.
Recent regulation such as the EU’s AI Act2 (see our Global AI Regulatory Tracker for more) is setting high ethical standards across Europe that affect multiple sectors, including the legal profession. These standards demand that AI systems be transparent, fair, and accountable,3 and they underscore the importance of legal professionals in interpreting and applying these rules.
Legal professionals must consider how biases in AI models could affect areas like hiring, credit assessments, or criminal sentencing. Recognising these implications is vital to guide clients in adopting AI responsibly, while proactively addressing potential ethical breaches. The deployment of AI in criminal justice4 and hiring processes5 illustrates how these challenges impact clients and communities directly.
These ethical concerns touch on fundamental issues, such as human rights, social justice, and the future of work. For a broader perspective on AI’s societal impact, see the recent thought-provoking essay by Anthropic CEO Dario Amodei, published in October 2024, which explores AI’s potential to shape society, for better or worse. These issues are especially critical in fields like law, healthcare, and government, where AI-driven decisions can profoundly affect individuals and communities.
Beyond advising clients, legal professionals have a role in AI policy discussions. By engaging in policymaking, establishing best practices, and advocating for comprehensive oversight, they help steer ethical AI development that upholds public interest and democratic principles. For today’s legal practitioners, AI ethics is not just a knowledge area but a cornerstone of responsible practice.
With the responsibilities of legal professionals in mind, we must now examine the key ethical challenges that arise in the implementation of AI systems across various sectors.
The Ethical Landscape of AI
AI regulation and policy are increasingly built upon core ethical principles, including human rights, justice, the rule of law, and democracy.
The EU has demonstrated that embedding core ethical principles—such as human rights, justice, the rule of law, and democracy—into AI development is essential for achieving trustworthy AI. UNESCO6 and the OECD7 have also championed these principles in their work on AI ethics, setting foundational standards that continue to guide other jurisdictions as they take their initial steps toward AI regulation.
These values frame the complex ethical challenges AI poses for societies, governance, and legal systems, such as:
- Accountability and Liability
- Key issue: Determining responsibility when AI systems cause harm or make errors can be complex.
- Legal challenge: Developing frameworks for AI liability and adapting existing tort and product liability laws to AI contexts.
- Privacy and Data Protection
- Key issue: AI systems often require vast amounts of data, raising concerns about data collection, storage, and usage.
- Legal challenge: Ensuring AI compliance with data protection regulations (e.g., GDPR, CCPA) and addressing issues of consent and data ownership.
- Autonomy and Human Oversight
- Key issue: As AI systems become more autonomous, questions arise about the appropriate level of human oversight and intervention.
- Legal challenge: Defining legal standards for human-in-the-loop requirements in critical AI applications.
- Intellectual Property Rights
- Key issue: AI-generated content raises questions about authorship, ownership, and patentability.
- Legal challenge: Adapting intellectual property laws to address AI-created works and inventions.
- Employment and Labor Rights
- Key issue: AI’s impact on the job market raises ethical concerns about displacement and the changing nature of work.
- Legal challenge: Balancing technological progress with worker protection and addressing issues of AI in the workplace.
- AI in the Legal System
- Key issue: The use of AI in legal processes (e.g., predictive policing, risk assessments in sentencing) raises ethical concerns about fairness and due process.
- Legal challenge: Developing guidelines for the appropriate use of AI in the justice system and ensuring constitutional protections.
- AI Safety and Security
- Key issue: Ensuring the safety and security of AI systems is crucial, particularly for high-stakes applications.
- Legal challenge: Developing safety standards and liability frameworks for AI systems, especially in critical sectors like healthcare and transportation.
These challenges are compounded by the ‘black box’ nature of AI systems, which obscures decision-making processes.
The “black box” challenge
The “black box” issue, is a central problem arising from AI. The term refers to the opacity of certain complex AI models that obscures how systems make decisions. This lack of transparency gives rise to critical legal and ethical issues when considering the increasing use of AI in sensitive fields like healthcare, finance, and criminal justice, where undetected biases or errors in AI systems may cause serious harm.
Another issue arising from this opacity, is the dilemma of transparency of technology vs the protection of trade secrets. In other words, how can the imperative for algorithmic transparency be achieved, whilst also protecting intellectual property and trade secrets. (This issue will be addressed further in a future issue of the Ethics Series)
As we navigate this complex terrain, our goal is to equip legal professionals with the knowledge and tools necessary to address these challenges effectively and ethically.
Brief explanation of the “black box” nature of AI
The “black box” nature of complex AI models with multiple neural lawyers, like deep learning, is a critical challenge for transparency and explainability. The terms arises because the internal workings are often hidden or too complex for clear interpretation,8 and thus how these systems reach their outputs can be opaque.
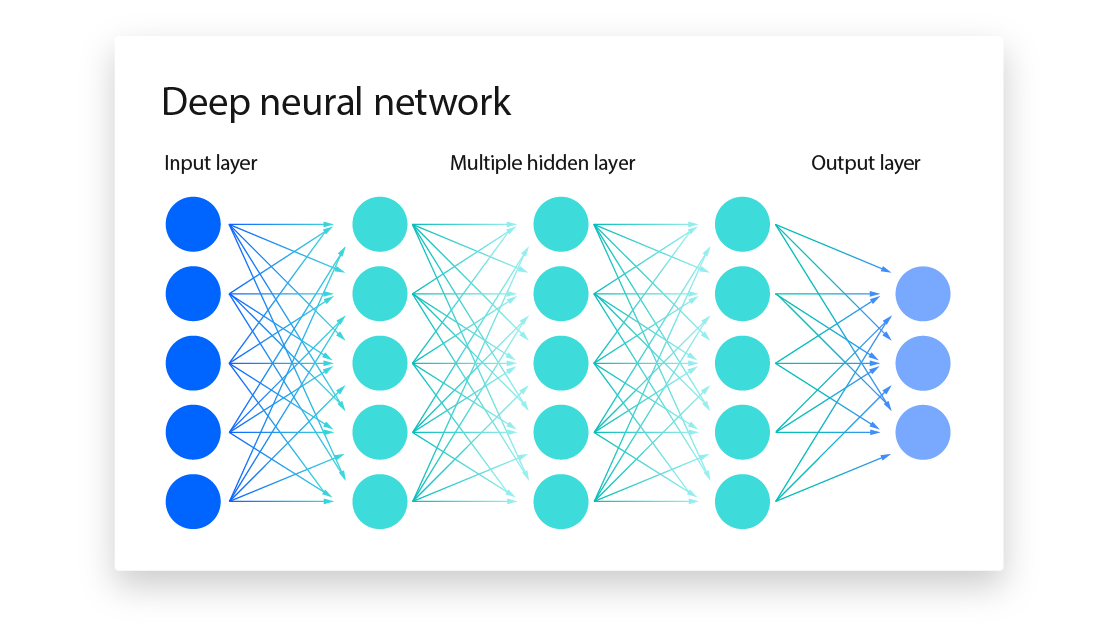
This issue is particularly pronounced in models with billions or even trillions of parameters (like GPT-4 reportedly has).9 Such models, critics argue, make it nearly impossible to trace back the decision path. This lack of interpretability raises both ethical and practical issues, particularly in sensitive fields such as healthcare, finance, and criminal justice.10
From an industry perspective, explainable AI (”XAI”) techniques are being developed to mitigate this problem. For example, methods such as the Local Interpretable Model-agnostic Explanations (”LIME”) and SHapley Additive exPlanations (”SHAP”) are commonly used to approximate how data influences model outputs.11 LIME creates simpler models around specific predictions, while SHAP uses game theory to quantify the importance of each feature in a prediction, allowing for both local and global insights into model behaviour. These techniques are crucial for improving transparency, although they provide only approximations of the complex internal processes of deep learning models.12
Academics have identified the “black box” issue as a central barrier to ethical AI, as it hinders efforts to ensure fairness, accountability, and reliability.13 Studies highlight that the opacity in these models restricts their adaptability, limits the ability to detect biases, and complicates error identification.14 This raises the stakes for integrating explainability into AI systems if they are to be trusted in real-world applications.
Recognising these challenges sets the stage for a critical exploration of bias and discrimination in AI, an area that demands urgent attention and action.
Bias and Discrimination in AI Systems
The subsequent article in the Deep Lex Ethics Series will open with an analysis of the issues arising from bias and discrimination in AI, including how new and evolving regulation may deal with this. In the meantime, we set out below an overview of the issues to be addressed.
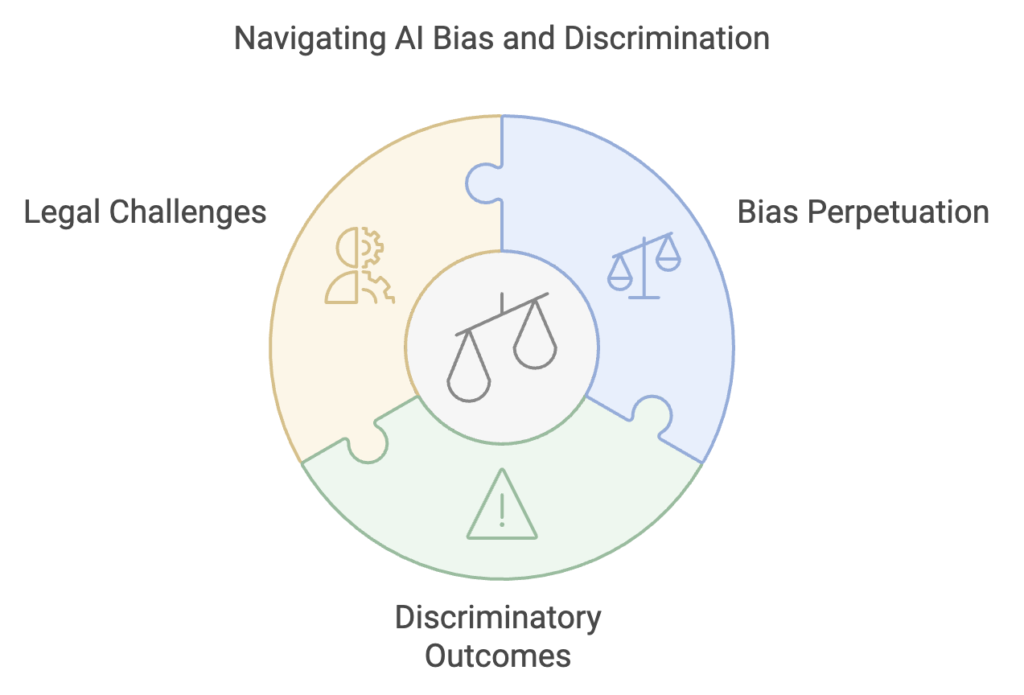
Here is why this issue is so urgent:
Issue 1: Bias in Training Data and Algorithms
- AI systems learn from data, which has been shown to reflect societal biases.15 For example, if a hiring algorithm is trained on historical hiring data from a company with a history of gender or racial bias, the AI could end up perpetuating these biases by unfairly favouring certain candidates over others. 16
- Algorithmic decisions can lack transparency, making it difficult for individuals to understand, let alone challenge, outcomes that feel unfair or discriminatory.
Issue 2: Impact on Human Rights and Equality
- AI used in surveillance, predictive policing,17 or credit scoring18 may disproportionately affect already vulnerable communities, reinforcing cycles of discrimination.
- In healthcare, biased AI systems could mean that minorities may receive lower-quality care due to misdiagnoses or inadequate treatment recommendations.19
Issue 3: Accountability and Transparency
- AI models are often complex and difficult to interpret, which creates challenges for accountability. If an AI system makes an incorrect or biased decision, key questions are raised as to who is responsible—whether it is the developers, the data providers, or the users of the AI.
- Also, a lack of transparency makes it hard to rectify errors or harm, especially when individuals suffer harm due to an AI system’s decision (see above regarding the “black box challenge”.
Issue 4: Scale and Speed of AI Deployment
- Because AI can be scaled quickly across industries, biased or unfair systems have the potential to affect large numbers of people before these issues are identified and addressed. This could create significant harm that may be challenging to reverse.
Issue 5: Autonomy and Control
- When AI systems make decisions with little human oversight, people may lose autonomy over key aspects of their lives. For instance, job applicants may be screened out of opportunities by an algorithm without understanding why.20
- This issue also affects democratic processes, demonstrated by AI-powered misinformation or micro-targeted political ads shown to be directed at manipulating public opinion and influencing elections.21 Such instances challenge individual autonomy on a societal level.
Efforts and Challenges in Addressing Bias and Ensuring Fairness
Efforts to address bias in AI are underway across academic, corporate, and regulatory spheres,22 but the pace of technological innovation often outstrips the development of ethical safeguards.
Researchers and developers are focusing on creating fairer algorithms utilising techniques like bias mitigation, model auditing, and fairness-aware machine learning.23 These techniques aim to identify and reduce bias in data and algorithms. For example, companies are now experimenting with de-biasing methods in hiring algorithms to ensure they do not discriminate against underrepresented groups.24
Global cooperation remains essential for ensuring AI promotes progress rather than perpetuating oppression. Ethics based guidelines and regulations, like the EU’s AI Act, hope to achieve this by advocating for fairness, accountability, and transparency. However, the real challenge appears to be for regulations and underlying ethics frameworks to keep pace with AI technology as it advances.
Preparing for an Ethical Future in AI
As AI transforms sectors across society, the importance of AI ethics becomes increasingly urgent, especially for legal professionals tasked with advising on responsible use and compliance. Addressing ethical issues such as bias, accountability, and transparency in AI is critical to protecting individual rights and ensuring that AI technologies support social progress rather than reinforce inequalities. Legal professionals, therefore, play a vital role in guiding clients through AI regulations and the requirements for AI to meet ethical frameworks.
To remain effective, lawyers must commit to ongoing education in AI ethics, staying informed about the latest regulatory developments and emerging best practices. Proactive engagement in policy discussions will further empower legal professionals to shape ethical AI policies that safeguard societal values.
We invite readers to subscribe to the Deep Lex newsletter for regular updates on AI ethics, regulation, and industry insights. By keeping informed and engaged, we can collectively work toward an AI future that balances innovation with responsibility, ensuring that AI serves as a force for positive change.
Disclaimer: The above is intended for information purposes only and does not constitute legal advice. Please refer to the terms and conditions page for more information.
Sources:
- Deep Lex, ‘The Impact of AI on the Law and Society’, 5 November 2024 ↩︎
- EU Artificial Intelligence Act (Regulation (EU) 2024/1689) ↩︎
- EU Artificial Intelligence Act (Regulation (EU) 2024/1689), see for example (27) ↩︎
- National Institute of Justice, “Using Artificial Intelligence to Address Criminal Justice Needs,” National Institute of Justice, 8 October 2018,(https://nij.ojp.gov/topics/articles/using-artificial-intelligence-address-criminal-justice-needs); Council on Criminal Justice, “The Implications of AI for Criminal Justice,” Council on Criminal Justice, accessed March 5, 2024, (https://counciloncj.org/the-implications-of-ai-for-criminal-justice/). ↩︎
- Jeff Winter, “Tech Recruiting: How To Leverage AI In The Hiring Process,” Forbes, June 2, 2023, (https://www.forbes.com/councils/forbesbusinesscouncil/2023/06/02/tech-recruiting-how-to-leverage-ai-in-the-hiring-process/). ↩︎
- UNESCO. “UNESCO Member States Adopt First Ever Global Agreement on Ethics of Artificial Intelligence.” UNESCO, November 25, 2021 (https://www.unesco.org/en/articles/unesco-member-states-adopt-first-ever-global-agreement-ethics-artificial-intelligence). ↩︎
- OECD, “The State of Implementation of the OECD AI Principles: Four Years On,” OECD Publishing, November 7, 2023 (https://www.oecd.org/en/publications/the-state-of-implementation-of-the-oecd-ai-principles-four-years-on_835641c9-en.html). ↩︎
- Samek, W., Wiegand, T., & Müller, K. R. (2017) “Explainable Artificial Intelligence: Understanding, Visualizing, and Interpreting Deep Learning Models” Cornell University (https://arxiv.org/abs/1708.08296); “AI’s Trust Problem,” Harvard Business Review, 3 May 2024 (https://hbr.org/2024/05/ais-trust-problem). ↩︎
- Josh Howarth, “Number of parameters in GPT-4 (latest data)”, Exploding Topics, 6 August 2024 (https://explodingtopics.com/blog/gpt-parameters). ↩︎
- Bender, E. M., Gebru, T., McMillan-Major, A., & Shmitchell, S. (2021). “On the Dangers of Stochastic Parrots: Can Language Models Be Too Big?” In Proceedings of the 2021 ACM Conference on Fairness, Accountability, and Transparency (pp. 610–623), Association for Computing Machinery (ACM),(version available here: https://dl.acm.org/doi/pdf/10.1145/3442188.3445922). ↩︎
- IEEE Xplore, “Peeking Inside the Black-Box: A Survey on Explainable Artificial Intelligence (XAI),” 2023 (https://ieeexplore.ieee.org/document/8466590). ↩︎
- EEE Xplore, “Explainable Artificial Intelligence: A Review and Case Study on Model-Agnostic Methods,” 2023, (https://ieeexplore.ieee.org/abstract/document/10373722). ↩︎
- Doshi-Velez, Finale, and Been Kim. “Towards a Rigorous Science of Interpretable Machine Learning.” Last modified February 27, 2017, (https://arxiv.org/abs/1702.08608). ↩︎
- Lipton, Zachary C. “The Mythos of Model Interpretability.” Communications of the ACM 61, no. 10 (2018), at pp. 36–43 (https://doi.org/10.1145/3233231). ↩︎
- Tiago P. Pagano et al, “Bias and Unfairness in Machine Learning Models: A Systematic Review on Datasets, Tools, Fairness Metrics, and Identification and Mitigation Methods” Big Data Cogn. Comput. 2023, 7(1), 15 (https://doi.org/10.3390/bdcc7010015). ↩︎
- As was the case for Amazon, Jeffrey Dastin, “Amazon Scraps Secret AI Recruiting Tool That Showed Bias Against Women,” Reuters, October 11, 2018 (https://www.reuters.com/article/world/insight-amazon-scraps-secret-ai-recruiting-tool-that-showed-bias-against-women-idUSKCN1MK0AG/). ↩︎
- Surveillance and Predictive Policing: Richardson, Rashida, Jason M. Schultz, and Kate Crawford. “Dirty Data, Bad Predictions: How Civil Rights Violations Impact Police Data, Predictive Policing Systems, and Justice.” New York University Law Review 94 (2019), at 192-233 (version available here: https://nyulawreview.org/online-features/dirty-data-bad-predictions-how-civil-rights-violations-impact-police-data-predictive-policing-systems-and-justice/). ↩︎
- Fuster, Andreas, Paul Goldsmith-Pinkham, Tarun Ramadorai, and Ansgar Walther. “Predictably Unequal? The Effects of Machine Learning on Credit Markets.” The Journal of Finance 77, no. 1 (2022), at 5-47 (https://papers.ssrn.com/sol3/papers.cfm?abstract_id=3072038). ↩︎
- Yang, J., Soltan, A.A.S., Eyre, D.W. et al. “Algorithmic fairness and bias mitigation for clinical machine learning with deep reinforcement learning.” Nat Mach Intell 5, 884–894 (2023) (https://www.nature.com/articles/s42256-023-00697-3). ↩︎
- Jeffrey Dastin, “Amazon Scraps Secret AI Recruiting Tool That Showed Bias Against Women,” Reuters, October 11, 2018 (https://www.reuters.com/article/world/insight-amazon-scraps-secret-ai-recruiting-tool-that-showed-bias-against-women-idUSKCN1MK0AG/). ↩︎
- World Economic Forum. “The Global Risks Report 2024.” World Economic Forum, January 2024, pp. 18-21 (https://www.weforum.org/publications/global-risks-report-2024/). ↩︎
- Kuhlman, Charles, MaryAnne Smart, and Rayid Ghani. “Learning to Address Race, Gender, and Intersectional Biases in AI Systems: A Human-Centered Approach.” Nature Machine Intelligence 5, no. 6 (June 2023) at 555-565; Pichai, Sundar. “AI at Google: Our Principles.” Google (blog), June 7, 2022 (https://blog.google/technology/ai/ai-principles/); EU Artificial Intelligence Act (Regulation (EU) 2024/1689), see for example (27). ↩︎
- Mehrabi, Ninareh, Fred Morstatter, Nripsuta Saxena, Kristina Lerman, and Aram Galstyan. “A Survey on Bias and Fairness in Machine Learning.” ACM Computing Surveys 54, no. 6 (July 2021) (https://arxiv.org/abs/1908.09635); Jeffrey Dastin, “Amazon Scraps Secret AI Recruiting Tool That Showed Bias Against Women,” Reuters, October 11, 2018 (https://www.reuters.com/article/world/insight-amazon-scraps-secret-ai-recruiting-tool-that-showed-bias-against-women-idUSKCN1MK0AG/); IBM also created, AI Fairness 360 an open source tool to “examine, report, and mitigate discrimination and bias in machine learning models throughout the AI application lifecycle” (https://aif360.res.ibm.com/) ↩︎
- Feldstein, Steven. “The Global Expansion of AI Surveillance.” Carnegie Endowment for International Peace, September 17, 2023 (https://carnegieendowment.org/2019/09/17/global-expansion-of-ai-surveillance-pub-79847). ↩︎